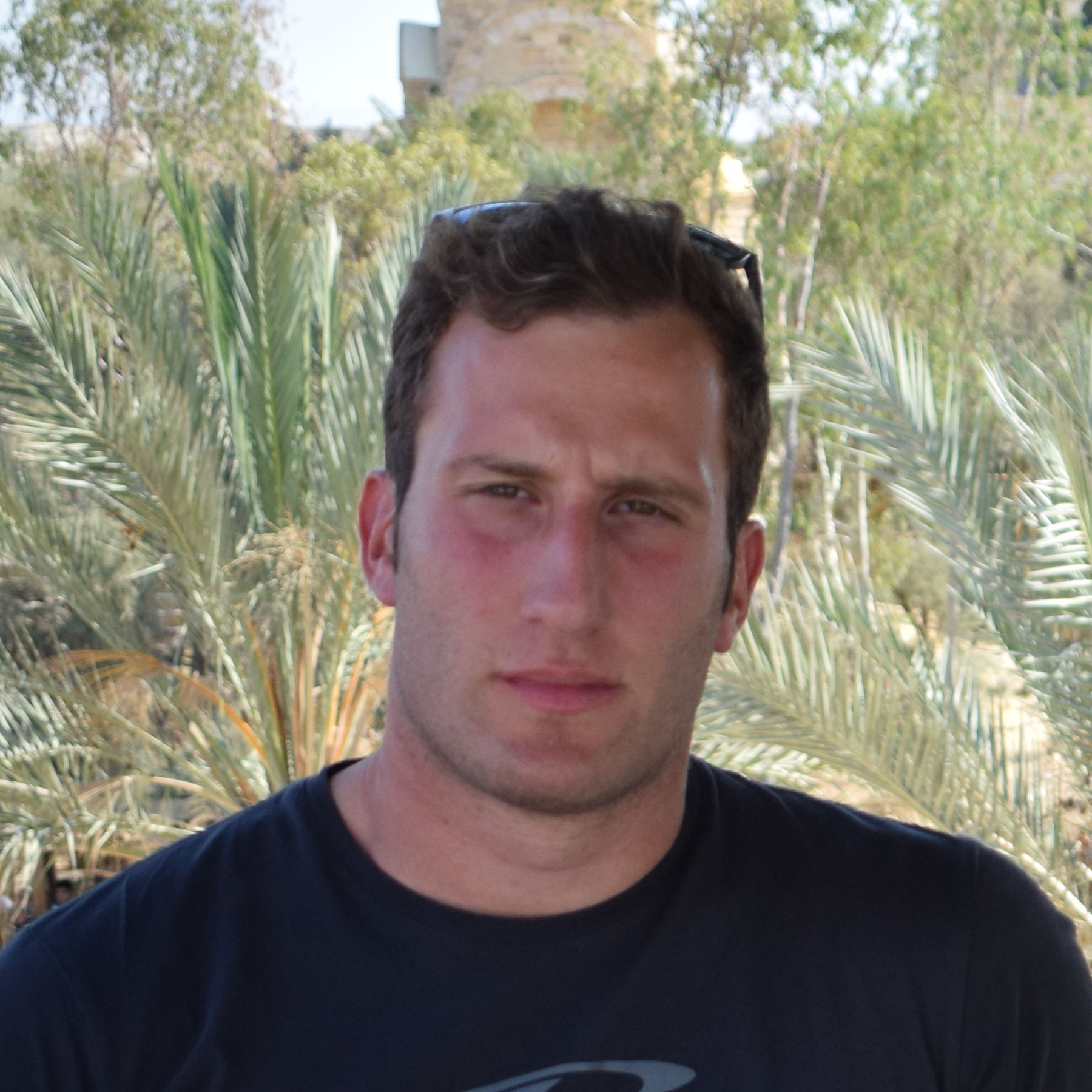
I am an ELLIS PhD student in the Computer Vision Lab at the University of Amsterdam, advised by Prof. Dimitris Tzionas. My research focus on 3D Human Object Interaction (HOI) synthesis, while I am also interested in reconstructing 4D HOIs from videos. Before joining UvA I had the great opportunity to spend 4 months as a research intern at Simon Fraser University working together with Prof. Manolis Savva. Prior to that I completed my Master at the University of Patras collaborating with Prof. Emmanouil Psarakis, while also working as a Lead Quality Assurance Enginner at Hellenic Air Force. I am also a passionate windsurfer. However, when the sea and wind are not there I enjoy spending my time running or going to the gym.
Publications
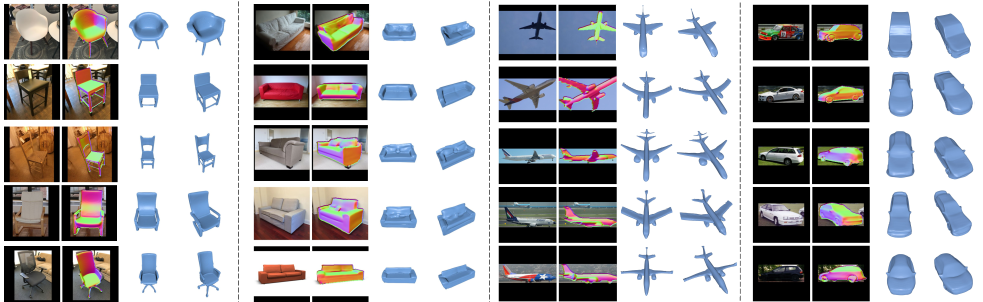
Recovering 3D object pose and shape from a single image is a challenging and highly ill-posed problem. This is due to strong (self-)occlusions, depth ambiguities, the vast intra- and inter-class shape variance, and lack of 3D ground truth for natural images. While existing methods train deep networks on synthetic datasets to predict 3D shapes, they often struggle to generalize to real-world scenarios, lack an explicit feedback loop for refining noisy estimates, and primarily focus on geometry without explicitly considering pixel alignment. To this end, we make two key observations: (1) a robust solution requires a model that imposes a strong category-specific shape prior to constrain the search space, and (2) foundational models embed 2D images and 3D shapes in joint spaces; both help resolve ambiguities. Hence, we propose SDFit, a novel optimization framework that is built on three key innovations: First, we use a learned morphable signed-distance-function (mSDF) model that acts as a strong shape prior, thus constraining the shape space. Second, we use foundational models to establish rich 2D-to-3D correspondences between image features and the mSDF. Third, we develop a fitting pipeline that iteratively refines both shape and pose, aligning the mSDF to the image. We evaluate SDFit on the Pix3D, Pascal3D+, and COMIC image datasets. SDFit performs on par with SotA methods, while demonstrating exceptional robustness to occlusions and requiring no retraining for unseen images. Therefore, SDFit contributes new insights for generalizing in the wild, paving the way for future research. Code will be released.
@article{antic2024sdfit, title={{SDFit}: {3D} {O}bject Pose and Shape by Fitting a Morphable {SDF} to a Single Image}, author={Dimitrije Antić and Sai Kumar Dwivedi and Shashank Tripathi and Theo Gevers and Dimitrios Tzionas}, journal={{arXiv}:2409.16178}, year={2024} }
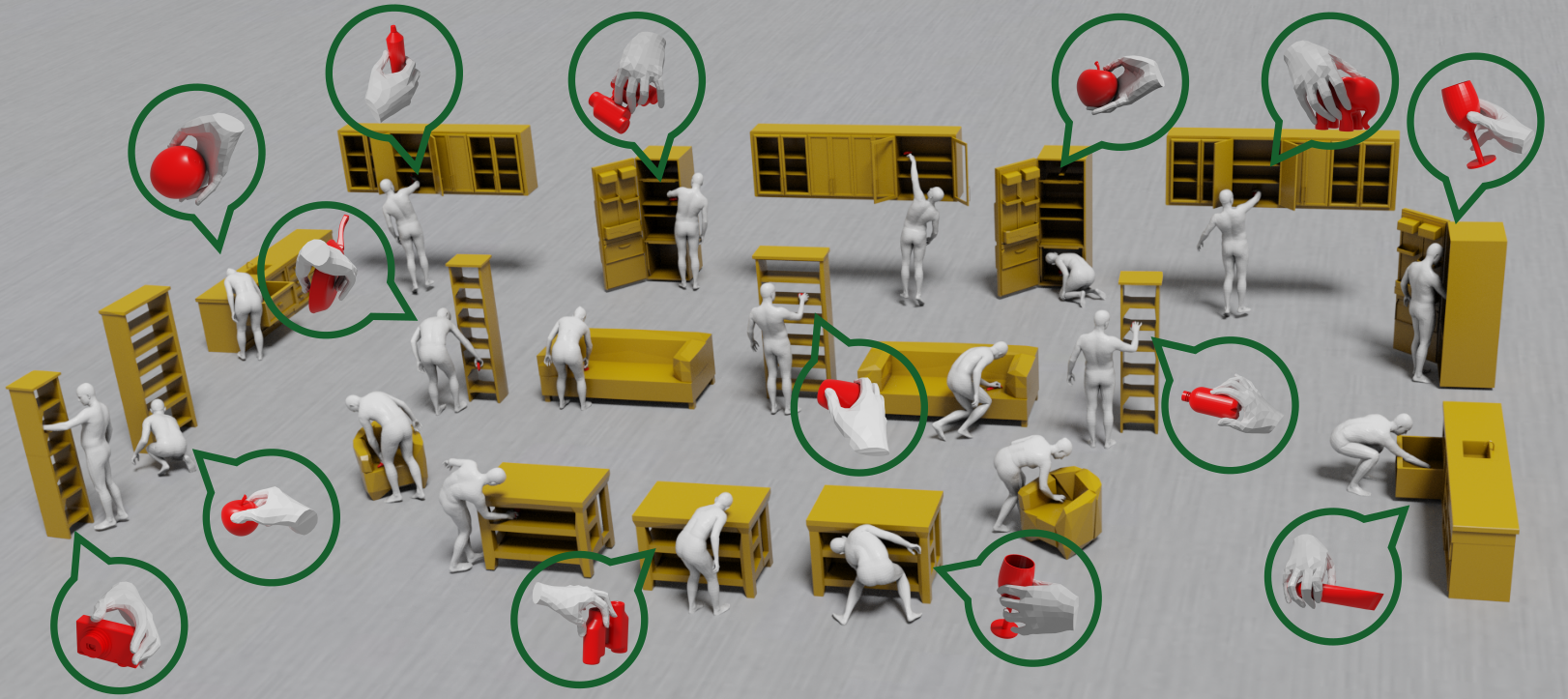
Synthesizing 3D whole bodies that realistically grasp objects is useful for animation, mixed reality, and robotics. This is challenging, because the hands and body need to look natural w.r.t. each other, the grasped object, as well as the local scene (i.e., a receptacle supporting the object). Moreover, training data for this task is really scarce, while capturing new data is expensive. Recent work goes beyond finite datasets via a divide-and-conquer approach; it first generates a “guiding” right-hand grasp, and then searches for bodies that match this. However, the guiding-hand synthesis lacks controllability and receptacle awareness, so it likely has an implausible direction (i.e., a body can’t match this without penetrating the receptacle) and needs corrections through major post-processing. Moreover, the body search needs exhaustive sampling and is expensive. These are strong limitations. We tackle these with a novel method called CWGrasp. Our key idea is that performing geometry-based reasoning “early on,” instead of “too late,” provides rich “control” signals for inference. To this end, CWGrasp first samples a plausible reaching-direction vector (used later for both the arm and hand) from a probabilistic model built via ray-casting from the object and collision checking. Then, it generates a reaching body with a desired arm direction, as well as a “guiding” grasping hand with a desired palm direction that complies with the arm’s one. Eventually, CWGrasp refines the body to match the “guiding” hand, while plausibly contacting the scene. Notably, generating already-compatible “parts” greatly simplifies the “whole”. Moreover, CWGrasp uniquely tackles both right and left-hand grasps. We evaluate on the GRAB and ReplicaGrasp datasets. CWGrasp outperforms baselines, at lower runtime and budget, while all components help performance. Code and models are available for for research.
@inproceedings{paschalidis2025cwgrasp, title={{3D} {W}hole-Body Grasp Synthesis with Directional Controllability}, author={Paschalidis, Georgios and Wilschut, Romana and Anti\'{c}, Dimitrije and Taheri, Omid and Tzionas, Dimitrios}, booktitle = {{International Conference on 3D Vision (3DV)}}, year={2025} }